Our blog
How to Enhance Splunk Data Model Acceleration?
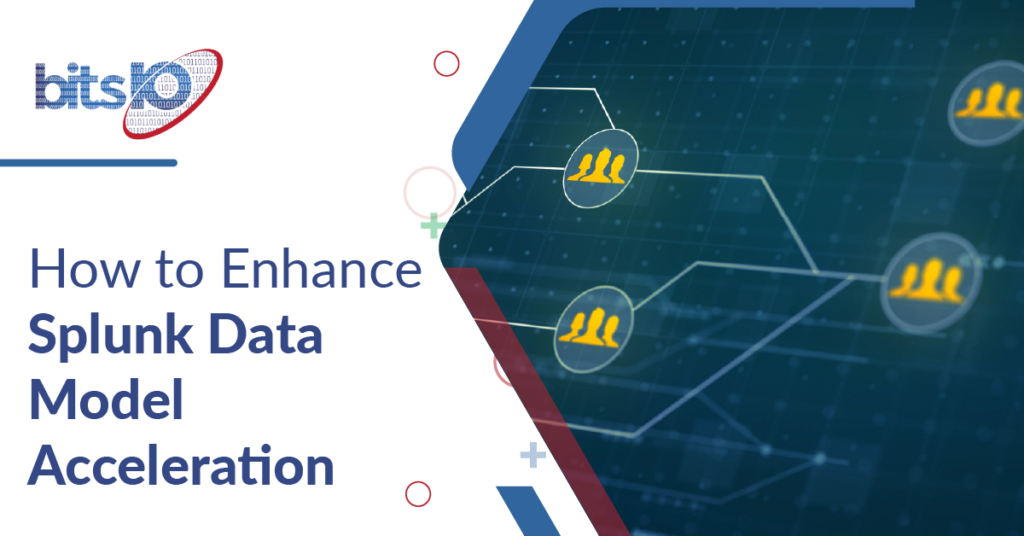
In the world of data analysis and cybersecurity, efficiency is the name of the game. You’ve invested in Splunk, a powerful tool for employing the insights hidden within your data. However, to truly maximize its potential, you need to ensure that your Splunk data models are operating at their peak performance.
Imagine a scenario where you can extract actionable intelligence from your data faster, with precision and reliability. This isn’t a far-fetched dream; it’s a reality that awaits you as we guide you to Accelerating Splunk Data Models: Boosting Performance with Proven Strategies.
Why Data Model Acceleration Matters
Before we dive into the strategies for enhancing Splunk Data Model Acceleration, let’s understand why it’s crucial. Splunk leverages Data Model Acceleration (DMA) to significantly boost the speed of searches compared to querying raw data. The need for DMA becomes particularly evident in products like Splunk Enterprise Security (ES), where continuous searches are conducted across substantial volumes of data. These searches aim to identify anomalies and security-actionable events promptly. To achieve this, ES primarily relies on running correlation searches against accelerated data models to yield rapid results.
So, how can you make the most of Splunk’s Data Model Acceleration feature?
- Â ConfigureAcceleration Settings:
Splunk provides various acceleration settings that can significantly impact DMA performance. Configuring these settings with the required parameters is essential to optimize acceleration. Here’s an explanation of some necessary settings:
Indexes Whitelist: The indexes whitelist is a crucial setting. By default, each data model searches all indexes, which can impact performance. To improve it, constrain the indexes that each data model searches. You can find more information about this setting in the Splunk documentation. - Change the Summary Range:
Another way to enhance DMA is by changing the summary range for data model accelerations. Adjusting the summary range can impact acceleration performance positively. You can find detailed information on how to do this in the Splunk documentation. - Utilize Splunk Search for DMA Information:
Splunk provides a powerful search query that gathers comprehensive information about Data Model Acceleration and its performance. This query helps you monitor and fine-tune DMA for optimal results.
Below is the Splunk search query for DMA information:
| rest /services/admin/summarization by_tstats=t splunk_server=local count=0
| eval key=replace(title,((“tstats:DM_” . ‘eai:acl.app’) . “_”),””), datamodel=replace(‘summary.id’,((“DM_” . ‘eai:acl.app’) . “_”),””)
| join type=left key
[| rest /services/data/models splunk_server=local count=0
| table title, “acceleration.cron_schedule”, “eai:digest”
| rename title as key
| rename “acceleration.cron_schedule” as cron]
| table datamodel, “eai:acl.app”, “summary.access_time”, “summary.is_inprogress”, “summary.size”, “summary.latest_time”, “summary.complete”, “summary.buckets_size”, “summary.buckets”, cron, “summary.last_error”, “summary.time_range”, “summary.id”, “summary.mod_time”, “eai:digest”, “summary.earliest_time”, “summary.last_sid”, “summary.access_count”
| rename “summary.id” as summary_id, “summary.time_range” as retention, “summary.earliest_time” as earliest, “summary.latest_time” as latest, “eai:digest” as digest
| rename “summary.*” as “*”, “eai:acl.*” as “*”
| sort datamodel
| rename access_count as “Datamodel_Acceleration.access_count”, access_time as “Datamodel_Acceleration.access_time”, app as “Datamodel_Acceleration.app”, buckets as “Datamodel_Acceleration.buckets”, buckets_size as “Datamodel_Acceleration.buckets_size”, cron as “Datamodel_Acceleration.cron”, complete as “Datamodel_Acceleration.complete”, datamodel as “Datamodel_Acceleration.datamodel”, digest as “Datamodel_Acceleration.digest”, earliest as “Datamodel_Acceleration.earliest”, is_inprogress as “Datamodel_Acceleration.is_inprogress”, last_error as “Datamodel_Acceleration.last_error”, last_sid as “Datamodel_Acceleration.last_sid”, latest as “Datamodel_Acceleration.latest”, mod_time as “Datamodel_Acceleration.mod_time”, retention as “Datamodel_Acceleration.retention”, size as “Datamodel_Acceleration.size”, summary_id as “Datamodel_Acceleration.summary_id”
| fields + “Datamodel_Acceleration.access_count”, “Datamodel_Acceleration.access_time”, “Datamodel_Acceleration.app”, “Datamodel_Acceleration.buckets”, “Datamodel_Acceleration.buckets_size”, “Datamodel_Acceleration.cron”, “Datamodel_Acceleration.complete”, “Datamodel_Acceleration.datamodel”, “Datamodel_Acceleration.digest”, “Datamodel_Acceleration.earliest”, “Datamodel_Acceleration.is_inprogress”, “Datamodel_Acceleration.last_error”, “Datamodel_Acceleration.last_sid”, “Datamodel_Acceleration.latest”, “Datamodel_Acceleration.mod_time”, “Datamodel_Acceleration.retention”, “Datamodel_Acceleration.size”, “Datamodel_Acceleration.summary_id”
| rename “Datamodel_Acceleration.*” as “*”
| join type=outer last_sid
[| rest splunk_server=local count=0 /services/search/jobs reportSearch=summarize*
| rename sid as last_sid
| fields + last_sid, runDuration]
| eval “size(MB)”=round((size / 1048576),1), “retention(days)”=if((retention == 0),”unlimited”,round((retention / 86400),1)), “complete(%)”=round((complete * 100),1), “runDuration(s)”=round(runDuration,1)
| sort 100 + datamodel
| table datamodel, app, cron, “retention(days)”, earliest, latest, is_inprogress, “complete(%)”, “size(MB)”, “runDuration(s)”, last_error
Here’s the Output:
The above output provides detailed information about only the accelerated data models. ‘Size (MB)’ indicates the amount of storage space consumed by the data model’s acceleration summary. ‘Run Duration (s)’ represents the duration of the summary search job. It’s important to note that ‘Run Duration’ is inversely proportional to performance.
A lower ‘Size (MB)’ and shorter ‘Run Duration’ correspond to higher performance, while a larger ‘Size (MB)’ and longer ‘Run Duration’ indicate lower performance.
Monitoring and Measurement
Effective monitoring and measurement are essential to ensure the success of your data model acceleration efforts. Splunk offers robust tools and techniques for tracking the performance of your accelerated data models.
Here’s a streamlined overview:
- Health Metrics: Use Splunk’s built-in metrics and dashboards to monitor your data model acceleration’s health. Keep an eye on metrics like completion status, search performance, and resource utilization.
- Alerting: Set up proactive alerts to detect any anomalies or issues in your acceleration process. Timely alerts help you address potential problems before they impact your security operations.
- Performance Baselines: Establish performance baselines for your accelerated data models. Monitor changes in search times, resource usage, and data model completeness. Any deviations from these baselines can signal optimization opportunities or potential issues.
- Capacity Planning: Regularly assess your infrastructure’s capacity to support data model acceleration without resource bottlenecks.
- User Feedback: Gather feedback from Splunk users and analysts who rely on accelerated data models. Their insights can provide valuable information about the effectiveness of your acceleration strategy.
- Continuous Improvement: Use monitoring data to identify areas for improvement. Adjust acceleration settings, constraints, and indexes based on performance trends and evolving data patterns.
Search Here
Latest Posts